Machine learning (ML) has become critical across multiple industries, from healthcare to finance. Understanding future trends and potential challenges is crucial for stakeholders as it grows in sophistication. It’s an exciting era for machine learning, as it promises to reshape how we solve problems and create opportunities. But with this transformation comes a host of ethical, technical, and practical challenges that must be addressed to harness its full potential effectively.
The Rise of Automated Machine Learning (AutoML)
AutoML aims to automate the more complex ML model building and deployment aspects. Companies are increasingly adopting AutoML platforms for their ease of use and efficiency. According to a recent article, AutoML technologies are expected to democratize machine learning, making it accessible to non-experts.
AutoML tools are designed to perform tasks such as data preprocessing, feature selection, and hyperparameter tuning automatically. It reduces the time required to build effective models and minimizes the need for specialized expertise. As these tools become more advanced, they will likely play a pivotal role in the widespread adoption of machine learning across various sectors. Businesses that previously couldn’t afford to invest in machine learning capabilities can now leverage their power through AutoML, thus democratizing the technology and fostering innovation at all levels.
Addressing Ethical Concerns in Machine Learning
Given the widening scope of ML applications, ethical concerns are mounting. Data privacy, algorithmic bias, and transparency are becoming hot topics. As highlighted in an article, the AI community increasingly emphasizes the importance of ethical guidelines to govern ML implementations.
Ethical guidelines are necessary to ensure that ML technologies are used responsibly. They help to maintain public trust and prevent potential misuse. Addressing ethical concerns also involves continuously evaluating ML systems to identify and mitigate biases. This proactive approach can significantly improve the accuracy and fairness of machine learning models. To ensure a broader societal benefit, the ethical use of machine learning must be a priority, foolproofing against privacy infringements and systemic biases and ensuring these technologies are inclusive and fair for all user groups.
Transformations in Healthcare Through Machine Learning
Machine learning is making remarkable strides in healthcare, particularly in predictive analytics and personalized medicine. ML models can now predict disease outbreaks and tailor treatments to individual patients, promising significant improvements in healthcare outcomes.
One notable application of machine learning in healthcare is in diagnostic imaging. Advanced ML algorithms can analyze medical images with a precision that rivals or surpasses human experts. Additionally, these models can detect patterns and anomalies that may be overlooked by traditional diagnostic methods, leading to earlier and more accurate diagnoses. Additionally, machine learning can help in drug discovery by identifying potentially therapeutic molecules quickly from large datasets, thus saving years of research and development time.
The Future of Work and Machine Learning
ML integration in workplaces transforms job roles and creates new opportunities. ML will completely change the nature of work by improving decision-making processes and automating repetitive jobs. However, this also comes with challenges related to workforce reskilling and maintaining job security.
As machine learning systems become more prevalent, employees must acquire new skills. Organizations must invest in training programs to help their staff adapt to the changing technological landscape. There is also an increasing focus on human-machine collaboration to attain the best results. By automating repetitive processes, this collaborative method can increase productivity and creativity by freeing up human workers to concentrate on strategic and inventive work.
Security Risks and Mitigation Strategies
As ML continues to evolve, so do the associated security risks. Malicious actors may exploit vulnerabilities in ML algorithms to their advantage. Therefore, robust mitigation strategies are crucial. Techniques such as adversarial training and anomaly detection can help safeguard ML models against these risks.
By exposing ML models to adversarial cases during training, adversarial training helps to strengthen their resistance to harmful inputs. Conversely, anomaly detection aids in spotting odd trends of behavior that could point to a security breach. Implementing these tactics may significantly increase machine learning systems’ resilience and security. Regular updates pat, patches, and continuous monitoring are essential practices that can help manage these risks effectively.
Open Research Problems and Their Impact
Despite significant advancements, numerous research challenges still need to be solved in machine learning. Issues such as model interpretability, data scarcity, and computational constraints are areas where further research could profoundly impact the future capabilities of ML.
One key challenge in machine learning research is ensuring that models are interpretable and transparent. While highly complex models like deep neural networks can achieve impressive results, their lack of interpretability can hinder their practical application. Developing methods to enhance the interpretability of these models is crucial for fostering trust and understanding among users. Additionally, addressing data scarcity through innovative data augmentation techniques and improving computational efficiency are critical areas of focus in the ongoing evolution of machine learning.
Conclusion
Machine learning has a bright future ahead of it, full of both potential and problems. By keeping up with current developments and addressing moral and security issues, stakeholders may fully utilize machine learning (ML) while reducing associated dangers. As research and development in this field continue to advance, the possibilities for machine learning applications are virtually limitless. Both people and businesses must take a proactive approach to tackling the inherent problems of this game-changing technology and keep up with evolving trends.
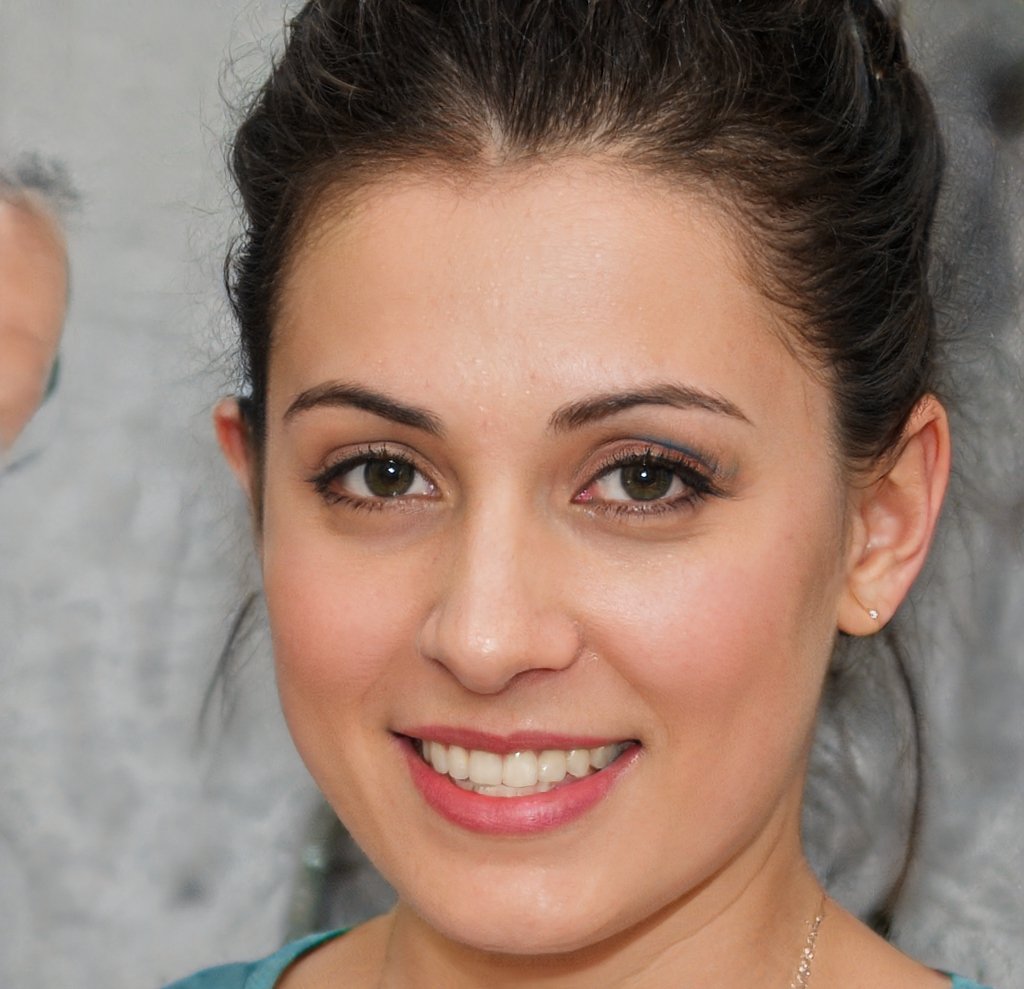
Laura Bennett is a vital member of The Jeep Diva team, handling all review and advertising inquiries. With a strong focus on the latest in celebrity news and entertainment, Laura ensures that each review is insightful and captivating. Her commitment to nurturing advertiser relationships keeps the blog lively and appealing. Outside of work, Laura loves discovering new media and engaging with readers to deliver unique and exciting content to The Jeep Diva audience.